When I explain how SEO works for Google, I’ll keep it to under 30 seconds for clients. Since I’ve done this, I have not put one client or prospect to sleep during a meeting.
Here’s what I’ll say: SEO is essentially a function of three umbrellas of factors: content on your website, offsite signals like links and citations, and the newer machine learning piece that tries to account better for what users are looking for.
(If their eyelids haven’t gotten heavy, I might explain a bit more.)
They’re calling it “rankbrain.”
Rankbrain is Google’s solution to the vast number of searches that have never before been seen.
You see, when people search “cars,” Google has, and almost always has had, tons of historical and real time data to determine the optimal results to serve those users.
But when people search, “what car is it that has the smiley face grill but not the one from mazda,” that’s a tougher problem.
Let’s pretend there is no direct existing data of past search volume on that phrase or necessarily even similar terms that Google could easily solve for in a split second through a cascading of algorithms based on grammar structure and nouns and however else search magic happens.
Enter “Google Hummingbird”
Hummingbird was an algorithm update targeted at answering this problem. It was based in machine learning and developed to solve for, “what does the user really want to know here?”
As a result, from about 2013 on we started to see knowledge cards and panels in Google results attempting to answer questions.
But that was just the beginning. These were in fact hundreds of thousands of little experiments collecting more training data to feed the beast.
Total aside: machine learning requires massive amounts of training data. In fact, even really bad machine learning projects see significant output improvements from just feeding it more and more data.
Context, user satisfaction, semantics, oh my
Hummingbird would account for lots of little intent signals: context, processing of similarly structured questions or phrases, metrics indicating user satisfaction with a search (like not having to perform more similar searches to massage the results).
Not that this was all made transparent; SEOs have picked up bits and pieces from a combo of reading between the lines of Google announcements, running our own experiments, and sharing findings in posts and on twitter.
But we do know a lot from understanding where machine learning is today in the context of history as well as what patents Google files (thanks to Bill Slawski.)
More than we should we also just sort of “guess.”
Semantic search considers the:
context of search, location, intent, variation of words, synonyms, generalized and specialized queries, concept matching and natural language queries to provide relevant search results.[2]
Tony John, via Wikipedia
Old school search used to look for keyword matches. New school search looks at synonyms, similar grammar patterns from previous searches, and more.
Anyhoo. Instead of showing you pictures of smiley faces, maybe some rappers smiling with gold and platinum grills, or suburbanites smiling while they barbeque with a new weber grill, it would instead show you exactly what you were looking for: car grills with a smiling shape.
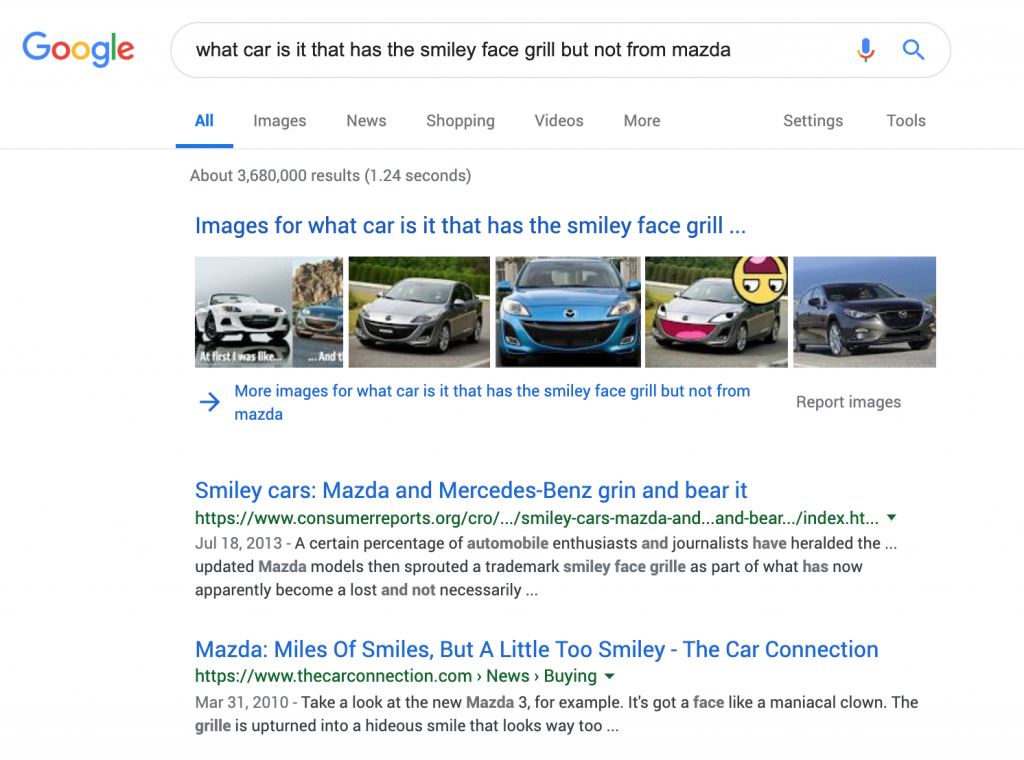
Think about it, how could you better solve for what your users want by paying more attention to the data, their context, what they say, what they really mean?
I came up with a pretty neat trick for reverse-engineering what users want using Google’s “people also ask” feature. You can use this to get quick and dirty ideas to write about.
And it’s four simple steps. Stay tuned for that next!
Leave a Reply